Support Vector Machines#
As a classifier, an SVM creates new dimensions from the original data, to be able to seperate the groups along the original features as well as any created dimensions.
The kernel that we choose tells us what constructed dimensions are available to us.
We will start with a linear kernel, which tries to construct hyper-planes to seperate the data.
For 2D, linearly separable data, this is just a line.
We use make_blobs
because it gives us control over the data and it’s separation; we don’t have to clean or standardize it.
Let’s make some blobs#
##imports
import numpy as np
import matplotlib.pyplot as plt
from sklearn import svm
from sklearn.datasets import make_blobs
X, y = make_blobs(n_samples = 100, n_features=2, centers=2, random_state=3)
## Plot Blobs
plt.scatter(X[:,0], X[:,1], c=y, cmap="viridis")
plt.xlabel(r'$x_0$'); plt.ylabel(r'$x_1$')
Text(0, 0.5, '$x_1$')
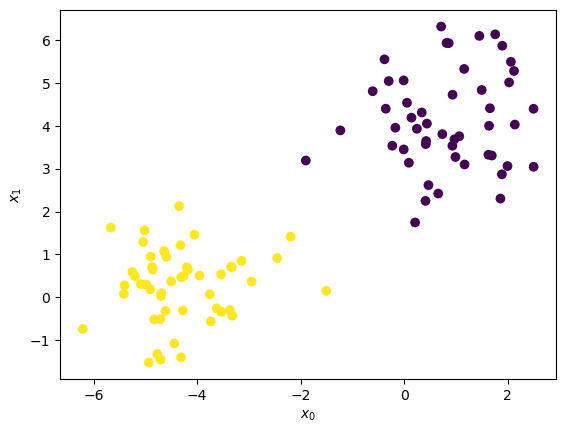
Let’s draw a separation line#
We are just guessing. SVM does this automatically.
## Make guess for separation line
plt.scatter(X[:,0], X[:,1], c=y, cmap="viridis")
xx = np.linspace(-6.5, 2.5)
#yy = -1*xx
#yy = -2 * xx - 1
yy = -0.5 * xx + 1
plt.plot(xx,yy)
[<matplotlib.lines.Line2D at 0x127c3a930>]
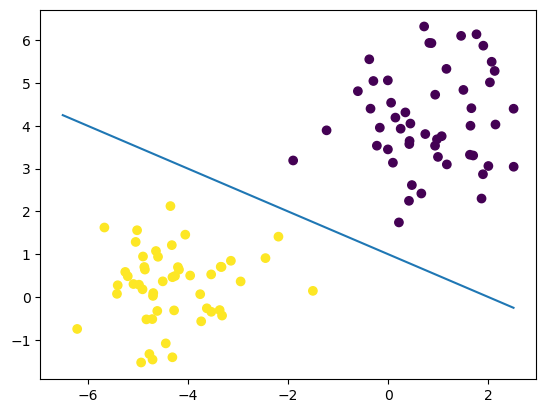