The Confusion Matrix#
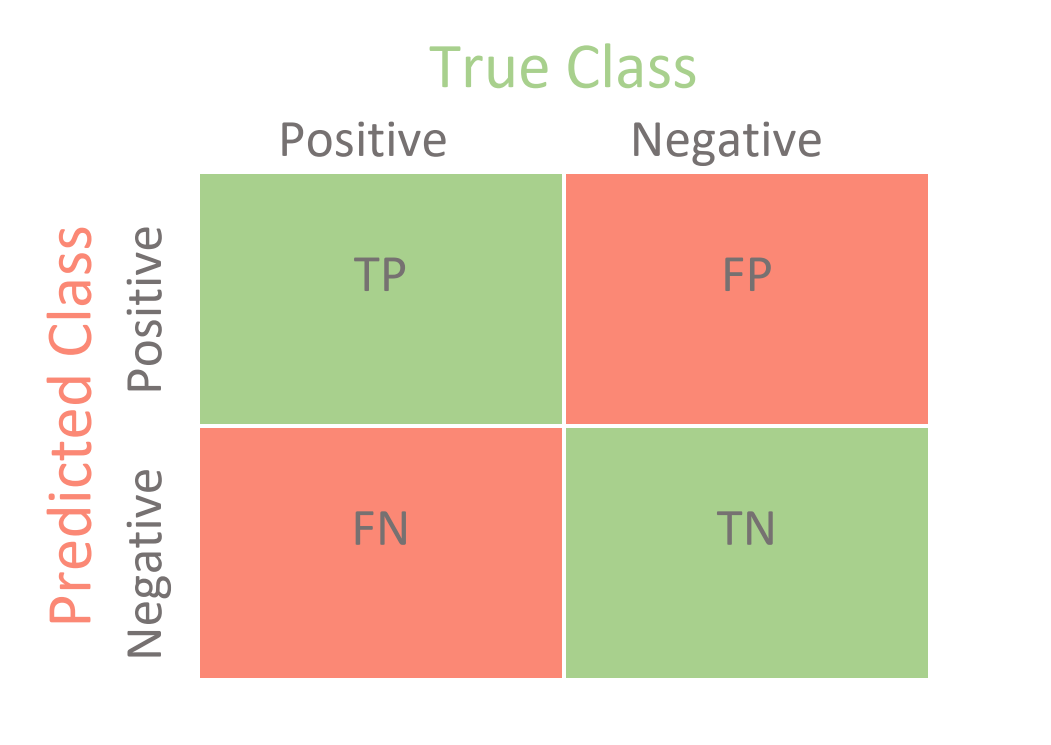
from sklearn.metrics import confusion_matrix
tn, fp, fn, tp = confusion_matrix(y_true, y_predicted)
Note the rows and columns of the confusion matrix from sklearn
do not match those show on most websites.
Other Metrics#
Sensitivity (Recall): The ratio of True Positives to all Positive Cases \(\dfrac{TP}{TP+FN}\)
Specificity: The ratio of True Negatives to all Negative Cases \(\dfrac{TN}{TN+FP}\)
Precision: The ratio of True Positives to all Predicted Positives: \(\dfrac{TP}{TP+FP}\)
\(F_1\) Score: A balanced measure (0 to 1) that includes sensitity and recall: \(\dfrac{2 TP}{2TP + FP + FN}\)
ROC Curve and AUC#
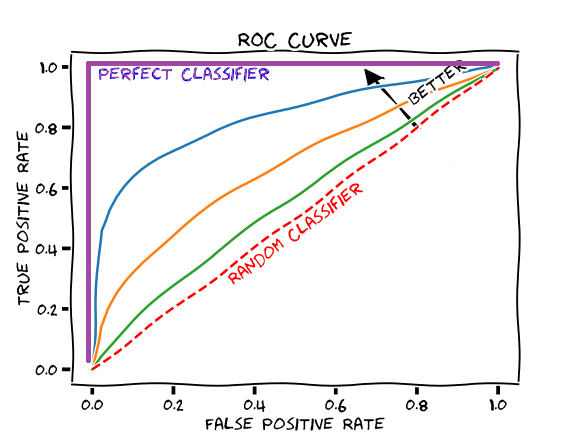
from sklearn import metrics
fpr, tpr, thresholds = metrics.roc_curve(y_true, y_predict)
roc_auc = metrics.auc(fpr, tpr)
plt.plot(fpr, tpr)
KNN as a Binary Classifier#
