Machine Learning#
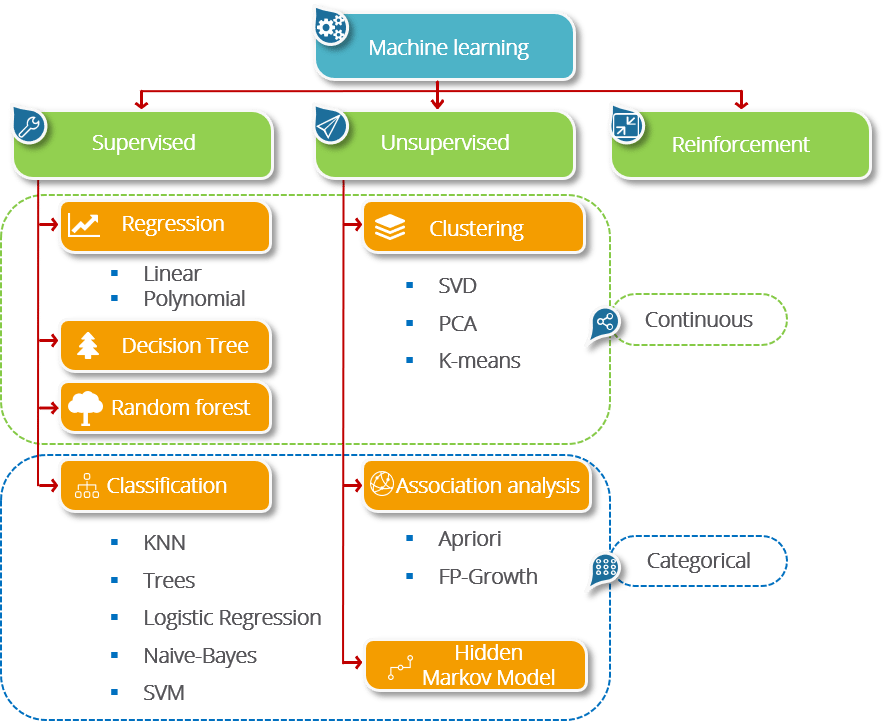
Classification#
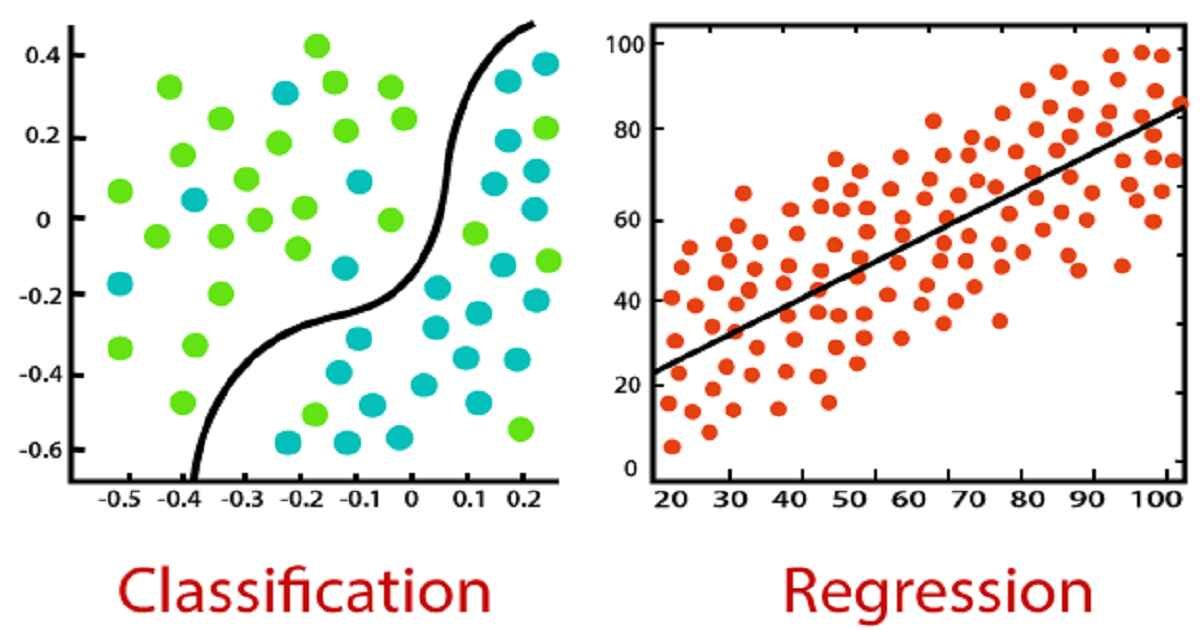
Classification Algorithms#
Logistic Regression: The most traditional technique; was developed and used prior to ML; fits data to a “sigmoidal” (s-shaped) curve; fit coefficients are interpretable
K Nearest Neighbors (KNN): A more intuitive method; nearby points are part of the same class; fits can have complex shapes
Support Vector Machines (SVM): Developed for linear separation (i.e., find the optimal “line” to separate classes; can be extended to curved lines through different “kernels”
Decision Trees: Uses binary (yes/no) questions about the features to fit classes; can be used with numerical and categorical input
Random Forest: A collection of randomized decision trees; less prone to overfitting than decision trees; can rank importance of features for prediction
Gradient Boosted Trees: An even more robust tree-based algorithm
We will learn Logisitic Regression, KNN, and SVM, but sklearn
provides access to the other three methods as well.
Generate some data#
make_classification
lets us make fake data and control the kind of data we get.
n_features
- the total number of features that can be used in the modeln_informative
- the total number of features that provide unique information for classessay 2, so \(x_0\) and \(x_1\)
n_redundant
- the total number of features that are built from informative features (i.e., have redundant information)say 1, so \(x_2 = c_0 x_0 + c_1 x_1\)
n_class
- the number of class labels (default 2: 0/1)n_clusters_per_class
- the number of clusters per class
import matplotlib.pyplot as plt
plt.style.use('ggplot')
from sklearn.datasets import make_classification
features, class_labels = make_classification(n_samples = 1000,
n_features = 3,
n_informative = 2,
n_redundant = 1,
n_clusters_per_class=1,
random_state=201)
print(features)
[[-0.25895345 0.43824643 -0.53923012]
[ 0.26877417 -0.52077497 0.54645705]
[ 0.45327374 -0.35995862 1.0255586 ]
...
[ 0.15015311 0.36829737 0.43754532]
[ 0.18243631 -0.28289479 0.38508262]
[ 0.33551129 1.46416089 1.10632519]]
print(class_labels)
[0 0 0 1 1 0 1 1 0 1 1 0 1 0 0 1 1 1 1 0 1 1 1 0 1 0 1 0 0 0 1 1 1 0 1 1 0
1 0 1 0 0 0 1 0 0 0 1 1 0 1 0 0 0 1 1 1 0 1 1 0 1 1 1 0 0 1 0 1 1 0 0 0 1
0 1 0 0 0 1 0 0 1 0 0 0 0 0 1 1 0 1 0 0 1 0 0 1 1 0 1 0 0 1 1 0 0 1 0 1 1
0 1 0 0 0 1 1 1 0 1 1 1 1 0 0 0 0 1 1 0 1 0 1 1 1 0 1 0 0 0 0 0 0 0 1 1 0
0 1 0 1 0 1 0 1 0 0 1 1 0 1 0 0 1 1 1 1 0 0 0 1 0 1 1 1 0 1 0 1 0 0 0 0 0
1 0 1 1 0 1 1 1 1 1 1 1 0 0 1 0 0 1 0 1 1 1 0 1 1 0 1 1 1 1 1 1 0 0 1 1 0
0 0 1 1 0 1 1 0 0 1 0 0 1 1 1 1 0 1 1 1 1 1 1 0 1 0 1 0 0 0 0 0 1 1 0 0 1
0 1 0 0 0 0 0 0 0 0 1 1 1 1 1 1 0 1 1 1 1 1 1 1 1 1 1 1 1 1 0 1 0 1 0 1 0
0 0 0 0 0 0 0 1 1 1 1 0 0 1 1 1 0 1 1 1 1 1 1 1 0 0 1 0 1 0 0 0 1 1 0 1 1
0 1 0 0 0 1 0 1 0 0 0 0 1 0 1 1 0 0 1 1 1 1 0 0 1 1 1 0 1 0 0 0 0 0 1 1 0
0 0 0 0 1 0 0 0 0 0 0 1 0 0 1 1 1 1 0 0 1 0 0 1 0 0 0 0 1 0 0 1 0 1 1 0 0
1 1 0 0 0 1 0 1 0 1 1 1 1 0 0 0 1 0 1 0 1 1 0 1 0 0 0 1 1 1 1 1 0 1 0 0 0
0 1 0 1 0 1 0 1 0 0 0 1 0 1 1 1 0 1 0 0 1 0 0 0 1 1 1 1 0 1 1 0 0 0 1 1 1
0 0 0 0 1 0 1 0 0 1 0 0 1 0 1 0 1 0 0 0 0 0 1 0 1 0 1 0 0 0 0 0 0 0 0 0 0
0 0 0 0 1 1 1 1 1 0 0 0 0 0 1 1 0 1 1 0 1 0 0 0 1 0 0 1 0 1 0 0 1 1 1 1 1
1 0 1 1 1 0 1 0 0 0 0 0 0 1 1 0 0 0 1 0 0 0 1 1 1 0 1 1 1 1 0 1 0 1 0 0 0
1 0 1 0 0 1 0 1 0 0 0 0 0 0 0 0 0 0 1 0 0 0 0 1 0 1 0 1 1 1 0 0 1 0 1 1 0
1 0 0 0 0 1 0 1 0 1 1 0 1 1 1 1 1 1 0 1 1 0 0 0 0 1 1 1 1 1 0 1 0 0 0 0 1
0 1 1 0 1 1 0 1 1 0 0 1 1 1 1 0 1 0 1 1 1 1 0 1 1 0 1 1 0 0 1 0 0 1 0 1 0
0 0 1 1 0 1 0 1 1 0 1 1 0 1 1 0 1 1 1 0 0 0 0 1 0 0 1 1 1 0 0 0 0 1 1 1 0
1 0 1 1 1 1 1 1 0 0 0 1 1 0 1 1 0 1 0 1 0 1 1 1 0 0 1 0 1 1 0 0 1 1 1 0 1
0 1 0 1 1 0 0 0 0 0 0 0 0 0 0 0 1 0 0 1 1 1 1 1 1 0 0 1 1 0 0 0 1 0 0 1 0
1 0 1 0 1 1 0 0 1 1 0 0 1 0 1 0 1 0 1 0 0 0 1 0 1 0 0 1 0 0 0 1 1 1 0 0 0
0 0 1 1 1 1 0 1 0 0 0 1 1 0 0 0 0 0 0 0 0 1 0 1 1 1 1 1 0 0 1 0 1 1 0 1 0
1 1 1 0 0 1 1 1 0 1 1 0 1 0 1 1 1 1 1 1 0 0 1 1 1 0 0 1 0 0 1 1 1 0 1 1 0
1 1 0 1 0 1 1 0 0 1 0 1 1 0 1 0 1 0 1 1 1 0 1 1 1 1 1 0 0 1 1 0 0 1 0 0 1
1 1 0 1 0 1 0 0 0 0 1 1 1 1 0 0 1 0 1 1 0 1 1 1 1 1 1 0 1 1 0 0 0 1 1 1 0
1]
## Let's look at these 3D data
from mpl_toolkits.mplot3d import Axes3D
fig = plt.figure(figsize=(8,8))
ax = Axes3D(fig, rect=[0, 0, .95, 1], elev=30, azim=135)
xs = features[:, 0]
ys = features[:, 1]
zs = features[:, 2]
ax.scatter3D(xs, ys, zs, c=class_labels, ec='k')
ax.set_xlabel('feature 0')
ax.set_ylabel('feature 1')
ax.set_zlabel('feature 2')
Text(0.5, 0, 'feature 2')
<Figure size 800x800 with 0 Axes>
## From a different angle, we see the 2D nature of the data
fig = plt.figure(figsize=(8,8))
ax = Axes3D(fig, rect=[0, 0, .95, 1], elev=15, azim=90)
xs = features[:, 0]
ys = features[:, 1]
zs = features[:, 2]
ax.scatter3D(xs, ys, zs, c=class_labels)
ax.set_xlabel('feature 0')
ax.set_ylabel('feature 1')
ax.set_zlabel('feature 2')
Text(0.5, 0, 'feature 2')
<Figure size 800x800 with 0 Axes>
Feature Subspaces#
For higher dimensions, we have take 2D slices of the data (called “projections” or “subspaces”)
f, axs = plt.subplots(1,3,figsize=(15,4))
plt.subplot(131)
plt.scatter(features[:, 0], features[:, 1], marker = 'o', c = class_labels, ec = 'k')
plt.xlabel('feature 0')
plt.ylabel('feature 1')
plt.subplot(132)
plt.scatter(features[:, 0], features[:, 2], marker = 'o', c = class_labels, ec = 'k')
plt.xlabel('feature 0')
plt.ylabel('feature 2')
plt.subplot(133)
plt.scatter(features[:, 1], features[:, 2], marker = 'o', c = class_labels, ec = 'k')
plt.xlabel('feature 1')
plt.ylabel('feature 2')
plt.tight_layout()
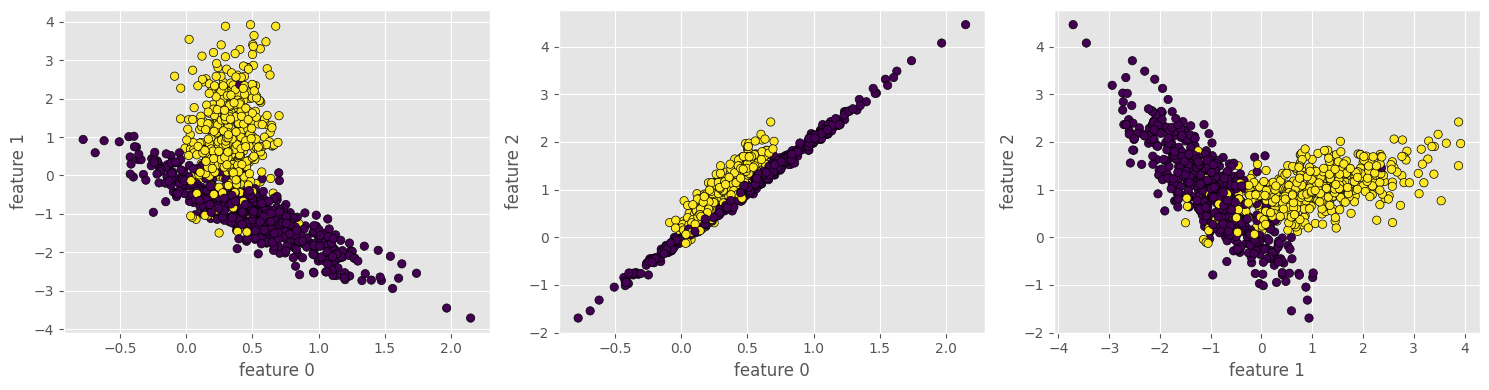
What about Logistic Regression?#
Logistic Regression attempts to fit a sigmoid (S-shaped) function to your data. This shapes assumes that the probability of finding class 0 versus class 1 increases as the feature changes value.
f, axs = plt.subplots(1,3,figsize=(15,4))
plt.subplot(131)
plt.scatter(features[:,0], class_labels, c=class_labels, ec='k')
plt.xlabel('feature 0')
plt.ylabel('class label')
plt.subplot(132)
plt.scatter(features[:,1], class_labels, c=class_labels, ec='k')
plt.xlabel('feature 1')
plt.ylabel('class label')
plt.subplot(133)
plt.scatter(features[:,2], class_labels, c=class_labels, ec='k')
plt.xlabel('feature 2')
plt.ylabel('class label')
plt.tight_layout()
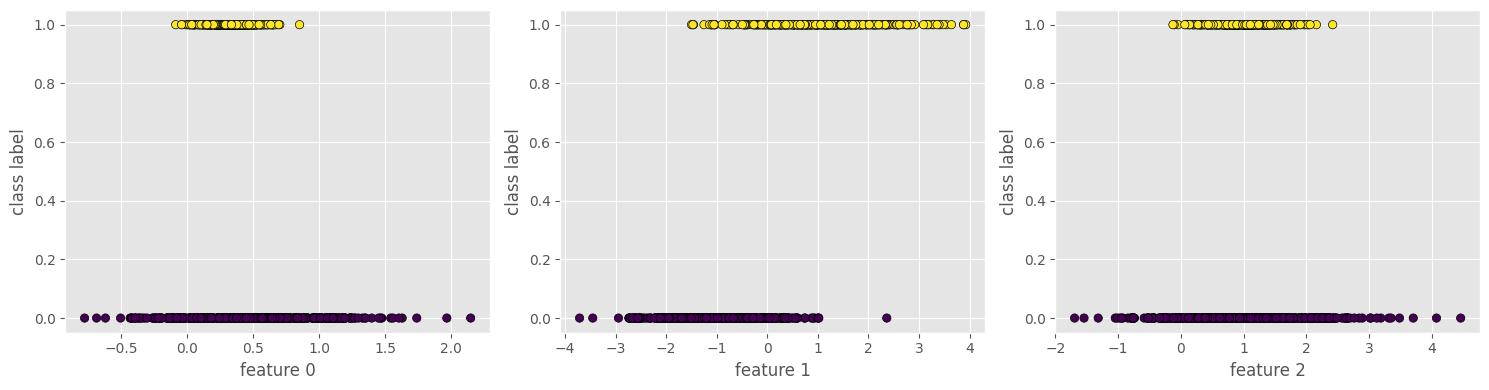